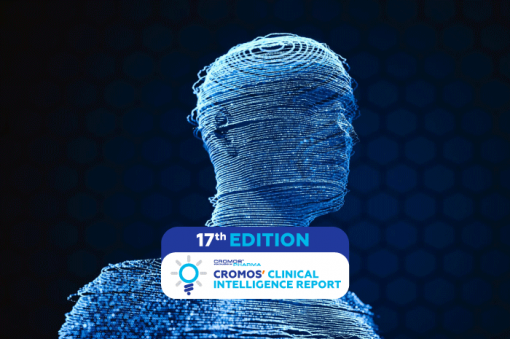
Digital Twins in Clinical Research: Revolutionizing Study Design
The concept of digital twins, a staple in industries like manufacturing and aerospace, is now making its way into the realm of clinical research. This technology involves creating a virtual replica of a physical entity—in this case, a patient or a biological process—which can then be used to simulate and predict outcomes without direct experimentation. For biotech professionals, digital twins hold the promise of transforming clinical trials, enhancing study safety, and optimizing therapeutic development. This article explores the potential of digital twins in clinical research, reviews their current state of development, and discusses the existing limitations.
The Rapid Growth of the Digital Twin Market
The global digital twin market is expanding rapidly, projected to grow from USD 17.73 billion in 2024 to USD 259.32 billion by 2032, with a compound annual growth rate (CAGR) of 39.8%. This impressive growth is driven by the technology’s ability to enhance efficiency, optimize processes, and reduce costs across various sectors.
Focusing specifically on clinical trials, the global healthcare digital twin market was valued at USD 1.17 billion in 2022 and is estimated to become a USD 38.43 billion market by 2032, growing at a staggering CAGR of 42.2%. The software segment dominated this market in 2022 with a significant 65.8% share. Within this space, the personalized treatment management application claimed a substantial 39.6% market share in 2022. Additionally, the surgical planning and risk assessment segment is projected to grow at an impressive CAGR of 19.7% from 2023 to 2032.
Potential of Digital Twins in Clinical Research
Digital twins are transforming clinical trials by offering unprecedented benefits. This technology enables researchers to create precise virtual models of patients or clinical environments, thereby enhancing the accuracy, efficiency, and safety of clinical trials. Here are some of the key ways digital twins are revolutionizing this field:
- Personalized Medicine. Digital twins allow for a highly personalized approach to treatment by simulating how different therapies might affect a specific patient based on their unique genetic profile, medical history, and lifestyle. This could drastically reduce trial and error in treatment selection, leading to faster, more effective trial outcomes.
- Enhanced Trial Safety and Efficiency. By predicting how patients might respond to a treatment before actual exposure, digital twins can help identify potentially harmful effects early in the process, thereby improving study safety. Furthermore, they can simulate different clinical trial scenarios, helping researchers to optimize study design, reduce costs, and shorten timelines.
- Predictive Maintenance in Clinical Settings. Just as digital twins in industrial settings are used to predict when machinery needs maintenance, in clinical research, they can predict when interventions might become necessary for patients in a trial, potentially preventing adverse events.
- Improving Patient Recruitment. Digital twins also facilitate patient recruitment by simulating trial participation. This can help address geographical and time commitment barriers, increasing patient engagement and compliance. Moreover, digital twins can be used to create a portion of the control group, ensuring more patients receive the experimental treatment, which can be a strong motivator for participation.
- Reducing Costs and Timelines. The use of digital twins can significantly reduce the costs and timelines of clinical trials. By avoiding the need for physical test subjects in early trial phases and automating data collection and analysis, sponsors can save both time and money. Digital twins can also help identify potential issues early on, preventing costly and time-consuming trial failures.
Current State of Development
Digital twins are becoming increasingly integral to clinical trials, significantly enhancing the simulation of drug interactions, prediction of patient outcomes, and refinement of trial protocols. Their adoption is driven by the integration of AI and machine learning, which bolsters their accuracy and utility, thereby enabling more precise and personalized treatment plans.
To create effective digital twins, it’s essential to integrate vast amounts of detailed, high-quality data, including genomic, proteomic, and phenotypic data. Advances in big data technologies and machine learning are gradually overcoming the challenges of data integration and management, enhancing the construction and usefulness of digital twins.
In terms of simulation and modeling technologies, there has been significant progress in developing sophisticated algorithms and computational models. These can simulate complex biological processes and drug interactions, although the biological complexity of humans presents challenges in predictive accuracy and real-world applicability.
On the regulatory and ethical front, frameworks are still evolving to keep pace with the rapid advancements in digital twin technology. There is a pressing need for clear guidelines on how these simulations can be utilized in clinical trials and decision-making processes. Regulatory bodies like the FDA and EMA are actively developing guidelines to ensure the accuracy, reliability, and ethical use of digital twins in clinical settings.
Ongoing Research and Trials
Several clinical trials are currently leveraging digital twin technology to validate its effectiveness and enhance precision treatments. Notable examples include:
- Type 2 Diabetes Treatment (NCT05181449): This ongoing trial uses digital twins to enable precision treatment for patients, improving the accuracy and effectiveness of therapeutic interventions.
- Strength Training for Musculoskeletal Conditions (NCT04849923): Another active trial using digital twins to optimize treatment plans for musculoskeletal injuries and diseases.
- Insulin Delivery and Meal Prediction Algorithms (NCT04203823): Completed trials have demonstrated the utility of digital twins in improving insulin delivery and predicting meal responses, thereby enhancing the management of diabetes.
Examples in Therapeutic Areas
- Oncology: Digital twins have been employed to simulate chemotherapy regimens for cancer patients. By using patient-specific data, researchers can identify the most effective and least toxic treatment combinations, significantly improving trial outcomes. For example, Altis Labs is collaborating with AstraZeneca and Bayer Pharmaceuticals to utilize AI-based digital twins in cancer trials, aiming to enhance the efficacy of treatments and accelerate clinical development (Definitive Healthcare) (Altis Labs | Home).
- Neurology: In clinical trials for neurological conditions like Alzheimer’s disease, digital twins help in predicting disease progression and treatment responses. The European Medicines Agency (EMA) has recognized the potential of digital twins in Alzheimer’s trials, supporting their use to improve trial efficiency and outcomes (Vanderbilt University).
- Cardiology: Digital twins are used to model cardiovascular conditions and predict responses to various interventions. This application helps in optimizing dosages and reducing adverse effects, thereby improving the safety and efficacy of cardiovascular treatments.
How Major Companies are Exploring Digital Twins
Innovative companies like Unlearn.AI are teaming up with industry giants such as Merck KGaA to incorporate digital twins into advanced-stage clinical trials, streamlining enrollment and accelerating drug development. The collaboration focuses on integrating prognostic data from Digital Twins into randomized controlled trials. This reduces the need for large control groups and speeds up regulatory approvals while maintaining accurate therapy effect estimates.
Unlearn.AI’s technology has proven it can reduce control arm sizes by 30% or more while generating reliable clinical evidence more quickly. In April 2020, Unlearn.AI raised $12 million in a Series A financing round to accelerate the use of its machine learning technology to enhance trial efficiency.
Regulatory Challenges for Digital Twins
Digital twins raise ethical and regulatory concerns, such as potential misuse of predictive capabilities. While some statistical methods are recognized by the FDA and EMA, the FDA lacks a dedicated qualification process and sufficient resources for thorough reviews. Although the FDA offers programs like the Complex Innovative Trial Design Meeting and Model-Informed Drug Development Paired Meeting, they are under-resourced. The EMA, being more conservative, has a specific pathway for reviewing software tools, funded by user fees, which the FDA lacks.
The successful use of digital twins heavily depends on available resources. Unlearn.AI received EMA qualification, showcasing the EMA’s supportive resources compared to the FDA’s limited capabilities, which may need Congressional support to improve. Ensuring the proper use of digital twins also involves addressing patient consent and data bias issues. Despite these challenges, regulators recognize the potential benefits of digital twins, considering their statistical reliability.
Limitations and Risks of Digital Twins in Clinical Trials
The potential of digital twins in clinical trials is immense, but realizing their full benefits involves overcoming several significant challenges and risks.
Digital twins require extensive patient data to create accurate representations. This data includes medical histories, genomics, and lifestyle factors. The complexity and volume of the data needed can be a significant barrier, especially for many healthcare organizations, limiting the effectiveness of digital twins unless they are used on a large scale.
Implementing digital twin technology requires substantial IT infrastructure and advanced equipment. The financial investment needed for hardware, software, and data storage is considerable, often making it feasible primarily for larger organizations with the necessary resources.
Another critical aspect is the security and privacy of patient data. The vast amounts of data processed by digital twins increase the risk of breaches. Implementing robust security measures is essential to protect sensitive information, which adds to the complexity and cost.
Finally, integrating digital twins into existing systems poses challenges in terms of interoperability and the need for continuous updates. These factors can complicate scalability and integration, hindering the widespread adoption of digital twin technology in clinical trials.
Digital twins are set to revolutionize clinical research by offering virtual replicas of patients or biological processes, enabling more personalized medicine, enhanced trial safety, and efficiency. Despite challenges such as data requirements, infrastructure costs, and regulatory compliance, the rapid growth of the digital twin market, particularly in healthcare, highlights its transformative potential. As technology advances and collaborative efforts continue, digital twins are poised to become integral to clinical research, improving patient care and accelerating therapeutic development.